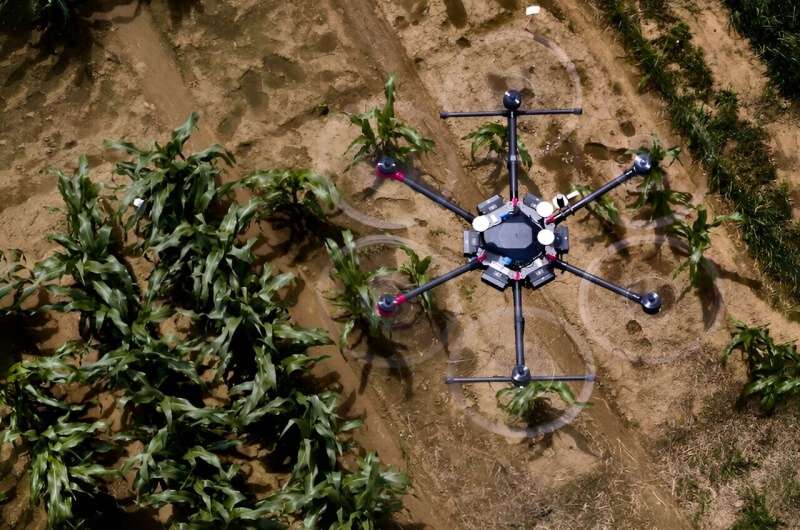
The software can visualize future plant growth using drone photos or other images from an early stage of growth. Credit: Plant methods (2024). DOI: 10.1186/s13007-024-01205-3
Researchers at the University of Bonn have developed software that can simulate the growth of agricultural crops. To do this, they fed thousands of photos from field experiments into a learning algorithm. This enabled the algorithm to learn how to visualize the future development of cultivated plants based on a single initial image. Using the images created during this process, parameters such as leaf area or yield can be accurately estimated.
Which plants should I combine in what ratio to achieve the greatest possible yield? And how will my production develop if I use organic manure instead of artificial fertilizers? In the future, farmers should increasingly be able to count on computer support when answering such questions.
Researchers have now taken a crucial step forward on the road to this goal. “We have developed software that uses drone photos to visualize the future development of the featured plants,” explains Lukas Drees from the Institute of Geodesy and Geoinformation at the University of Bonn. The early career researcher is an employee at the PhenoRob Group of Excellence.
The large-scale project based at the University of Bonn aims to advance the smart digitization of agriculture to help agriculture become more environmentally friendly without causing crop yields to suffer. The findings are published in the journal Plant methods.
A virtual look into the future to aid decision-making
The computer program now presented by Drees and his colleagues is an important building block. It should eventually make it possible to virtually simulate certain decisions – for example, to assess how the use of pesticides or fertilizers will affect crop yields.
For this to work, the program must be fed drone photos from field experiments. “We took thousands of images over a period of growth,” explains the PhD researcher. “In this way, for example, we documented the development of cauliflower crops under certain conditions.
The researchers then trained a learning algorithm using these images. Then, based on a single aerial image of an early growth stage, this algorithm was able to generate images showing the future development of the crop in a new, artificially created image.
The whole process is very accurate as long as the crop conditions are similar to those present when the training photos were taken. Consequently, the software does not take into account the effect of a sudden cold snap or steady rain lasting several days. However, it needs to learn in the future how growth is affected by such influences, as well as the increased use of fertilizers, for example. This should enable him to predict the outcome of certain interventions by the farmer.
“In addition, we used a second AI software that can estimate different parameters from plant photos, such as crop yield,” says Drees. “This also works with generated images. Thus it is possible to precisely estimate the subsequent size of cauliflower heads at a very early stage of the growing period.”
Focus on polycultures
One area where researchers are focusing is the use of polycultures. This refers to planting different species in one field – such as beans and wheat. Since plants have different requirements, they compete less with each other in a polyculture of this type compared to a monoculture, where only one species is grown. This increases yield. In addition, some species – beans are a good example of this – can fix nitrogen from the air and use it as a natural fertilizer. Other species also benefit from this, in this case wheat.
“Polycultures are also less susceptible to pests and other environmental influences,” explains Drees. “However, how well this all works depends very much on the species combined and their mixing ratio.”
When the results from many different mixing experiments are fed into learning algorithms, it is possible to make recommendations about which plants are particularly compatible and in what ratio.
Plant growth simulations based on learning algorithms are a relatively new development. Process-based models have been mainly used for this purpose so far. These – metaphorically speaking – have a basic understanding of the nutrients and environmental conditions that certain plants need during their growth in order to thrive.
“Our software, however, only makes its statements based on the experience they’ve gathered using the training images,” Drees points out.
Both approaches complement each other. If they were combined in the right way, it could significantly improve the quality of predictions. “This is another point that we are investigating in our study”, says the doctoral researcher. “How can we use process-based and image-based methods so that they benefit from each other in the best possible way?”
More information:
Lukas Drees et al, Simulation of data-driven crop growth on time-varying generated images using multi-conditional generative adversarial networks, Plant methods (2024). DOI: 10.1186/s13007-024-01205-3
Provided by the University of Bonn
citation: Artificial intelligence shows how crops develop in the field: Software can simulate future growth based on a single initial image (2024, June 17) Retrieved June 18, 2024 from https://phys.org/news/2024 -06-ai-field-crops-software-simulate.html
This document is subject to copyright. Except for any fair agreement for study or private research purposes, no part may be reproduced without written permission. The content is provided for informational purposes only.
#Artificial #intelligence #shows #crops #develop #field #Software #simulate #future #growth #based #single #initial #image
Image Source : phys.org